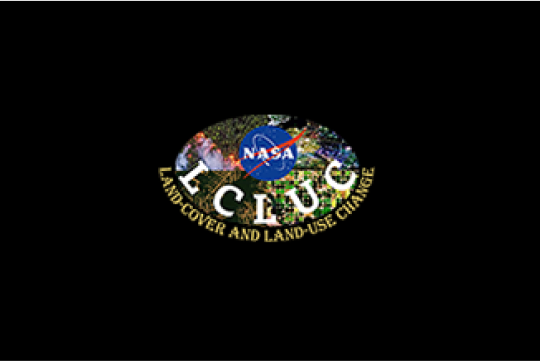
Team Members:
Person Name | Person role on project | Affiliation |
---|---|---|
Marc Simard | Principal Investigator | Caltech/ Jet Propulsion Laboratory, Pasadena, United States |
Temilola Fatoyinbo | Co-Investigator | |
Victor Rivera | Co-Investigator | |
Rinku Roy Chowdhury | Co-Investigator |
Mangrove forests are coastal wetlands that contribute to regional, continental and global biodiversity and act as major biogeochemical links between upland and coastal regions of the tropics. Mangrove ecosystems provide a great number of ecosystem services ranging from shoreline protection (e.g. against erosion, tsunamis and storms, nutrient cycling, fisheries production, lumber and habitat). But as a result of their location and economic value, they are among the most rapidly changing landscapes. The greatest current threats to mangroves derive from human activities such as aquaculture, freshwater diversions, overharvesting and urban and industrial development. The effects of sea-level rise (SLR) and increased extreme climatic events may also increase the vulnerability of this ecosystem to global change. Our objective is to develop spatially explicit models of mangrove forest vulnerability to anthropogenic activity and climate change across the Americas. We propose a multi-scale nested approach with continental-scale mapping of mangroves and land cover land use (LCLU) for the entire Americas, regional-scale analyses in 18 sites, and in-depth studies in 7 local sites. These models will be produced by integrating socioeconomic datasets and local surveys with multi-sensor remote sensing of mangrove use and cover change, and eco-geomorphology. We propose a novel LCLU classification scheme that will include proximate sources of mangrove conversion (e.g. shrimp farming, logging, agricultural expansion, hydrological modifications leading to die-offs, growth of urban areas/fronts, etc.), and mangrove forest structural classes (e.g. height). We will use a combination of optical (i.e. Landsat) and radar (i.e. ALOS/PALSAR, JERS-1,UAVSAR, SRTM) timeseries data as well as lidar (ICESat/GLAS) to cover all mangrove regions of the Americas. These data will also be used to map canopy height and geomorphology. Importantly, canopy height will enable us to assess the potential services associated to different canopy structures and thus, their vulnerability to various drivers of change. Coastal geomorphology is a significant environmental determinant of mangrove structure, and influences mangrove resilience to SLR. But, SLR resilience also depends on soil accretion. Sediment deposition (inorganic) and biotic processes such as root production (organic) contribute to soil volume in mangrove forests, allowing these wetlands to keep up with SLR. Within the 7 local sites, we will measure the rate of soil accretion and develop potential relationships with canopy structure and geomorphology to upscale to the Americas. Geomorphology and accretion rates will be compared to local estimates of SLR to derive local vulnerability indexes to SLR. The remote sensing studies of mangrove regions will be coupled with commensurate, extensive ecological and social (census) datasets for 18 regions, and used to develop regional-scale models of mangrove vulnerability. Moreover, intensive assessments will be conducted for calibration and validation of the regional-scale models, relating socioeconomic activity with local changes in mangrove forest use and cover. These intensive assessments will focus on a set of 7 coastal mangrove study regions representing a wide range of socio-demographic, economic, policy and ecogeomorphic contexts found throughout the Americas. These sets of multi-scale assessments will enable upscaling of regionally calibrated models and the generation of continental scale, spatially explicit scenarios of mangrove vulnerability using widely available socioeconomic and remote sensing data products.