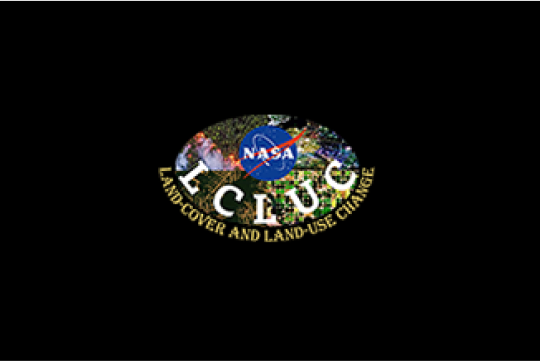
Team Members:
Person Name | Person role on project | Affiliation |
---|---|---|
Michael Keller | Principal Investigator | USDA Forest Service, Jet Propulsion Laboratory, Pasadena, USA |
Tropical forests account for more than half of terrestrial aboveground carbon stocks. They are extremely biodiverse, hosting 60–70% of terrestrial species despite covering only 7–10% of the land and are critical to biodiversity conservation. The future terrestrial carbon budget is highly uncertain according to Earth system models largely because of uncertainties related to the future of tropical forests. Deforestation and degradation lead to losses of tropical forest carbon and biodiversity. However, the second growth and degraded forests that regenerate after disturbance represent potential sinks for carbon and refuges for biodiversity. Second growth and degraded forests almost certainly cover more area today than intact forests. The Amazon region of Brazil, a hotspot for deforestation for nearly half a century, is now home to large extents of second growth and degraded forests. These forests are important to Brazil’s plans to mitigate greenhouse gas emissions. Second growth forests in the Brazilian Amazon are well mapped in contrast to degraded forests that are the subject of much ongoing research, including our own. We will focus on improving the classification of degraded forests through the incorporation of thermal data into our developing classification approach. Additionally, we will incorporate thermal data in multisource remote sensing to determine how drought and fire limit the regeneration capacity of secondary and degraded forests. We propose to use Multi-Source Land Imaging methods combining infrared data (both shortwave infrared (SWIR) and thermal infrared (TIR) with passive and active (lidar) optical data and models of forest growth to study second growth and degraded forests in the Brazilian Amazon, a hotspot of land use change. Our planned study has three objectives: (1) To improve classification of degraded forests across the Brazilian Amazon by combining data from thermal remote sensing with a previous texture analysis from multispectral passive optical remote sensing; (2) To understand the temperature and moisture controls on second growth forest biomass accumulation through models of these controls that predict where forests are likely to flourish and where they are likely to stagnate or die-off; (3) To understand the temperature and moisture controls on the regeneration of degraded forests where the starting point for forest recovery is poorly constrained. Our study will improve our understanding of land-cover and land-use change (LCLUC) by enhancing our ability to detect and quantify the extent of degraded tropical forest (Obj. 1). Additionally, we will improve our ability to predict whether second growth and degraded forests will expand on the landscape or whether they will be more susceptible to drought and fire mortality (Obj. 2 & 3). Our study will go beyond identifying the presence of second growth forests on contemporary and historical landscapes, to understanding the conditions that will either promote the growth and proliferation of those forests or lead to their stagnation or disappearance. The work will rely on thermal remote sensing (ECOSTRESS - ECOsystem Spaceborne Thermal Radiometer Experiment on Space Station, MODIS - Moderate Resolution Imaging Spectroradiometer, and VIIRS - Visible Infrared Imaging Radiometer Suite), multisource inputs (passive optical Sentinel-2, airborne lidar, GEDI - Global Ecosystem Dynamics Investigation lidar), and open-source data products. We will rely on machine learning classification models to accomplish task 1 and on hierarchical Bayesian models for tasks 2 and 3. Our research will result in improved classification of degraded forests in the Brazilian Amazon. Our models of secondary and degraded forest growth as influenced by moisture availability and fire disturbance history will provide us with understanding of the future forest landscape of the Brazilian Amazon.