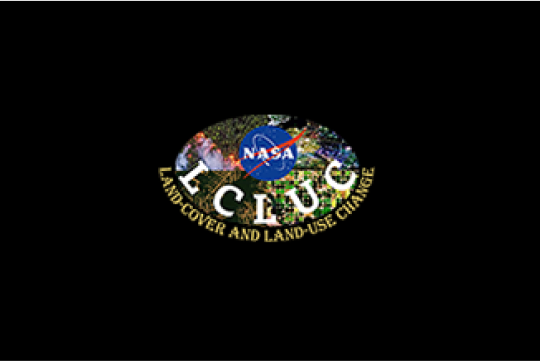
Team Members:
Person Name | Person role on project | Affiliation |
---|---|---|
Yufang Jin | Principal Investigator | UC Davis, Davis, USA |
Yong Jae Lee | Co-Investigator | University of Wisconsion-Madison, Madison, USA |
Yuhan Huang | Graduate Student Researcher | University of California, Davis, Davis, USA |
Yuheng Li | Graduate Student Researcher | UW-Madison, Madison, USA |
Wildland–Urban Interface (WUI), a transition zone between unoccupied wilderness and human settlement, is a critical land cover and land use change “hotspot”. Its rapid growth in the US has disrupted the ecology, e.g., increasing wildfire risk and the associated socio-economic consequences, wildlife habitat loss, and degradated water quality. The most widely used WUI datasets have been heavily relying on the decadal block-level census data, although some attempts have made to incorporate the 30m vegetation cover from Landsat observations and night time light data at a coarser resolution. A more detailed WUI products with key attributes, such as footprints of buildings/roads and human modification on fuels at a finer scale, are needed for city planners, disaster responders, and fire managers.
This project aims to integrate a suite of multi-spectral satellite imagery at moderate (e.g., Landsat, Sentinel-2) to very high resolution (e.g., RapidEye and PlanetScope), and radar, lidar and hyperspectral satellite imagery (Sentinel-1, GEDI, and PRISMA), with other aerial imagery and social “big” data, such as OpenStreetMap (OSM) and county data. We will develop convolutional neural network (CNN) based data fusion approaches to combine complementary information from these multi-source and multi-scale data. Advanced deep learning computer vision algorithm, i.e., fully convolutional network, will be applied for semantic segmentation and combined with YOLOACT instance segmentation, to identifyindividual objects of buildings/roads and trees/shrubs at the scale of 1 to 5 meters. The verified OSM labels and annotated ImageNet database will be used for training and testing. The snapshots of Census block data will be used as a constraint for our fine resolution mapping. We will also further characterize the attributes of buildings, vegetation, and other impervious surfaces by fusing structural and hyperspectral-features. These fine grained housing and vegetation information will be further used to generate improved WUI maps for the baseline years. We will then apply a change detection and targeted prediction framework to update the maps annually in a scalable way.
Our overall goal is to developing robust deep learning approaches to detect and characterize fine grained human settlements and vegetation for improved annual WUI mapping, and implementing a scalable workflow with transfer learning for regional applications. The key deliverables include (1) a set of scalable open source tools and workflow for feature-level data fusion of multi-source imagery, object identification with VHR imagery, and change detection, and etc.; 2) baseline and annual maps of fine grained footprints of buildings, roads, trees/shrubs, and other vegetation type within the WUIs; (3) Annual semantic maps of key attributes about the unique WUI fuels including buildings and vegetative fuels; (4) Improved annual maps of WUI and the associated characteristics ]and (5) case study results about the impact of human development patterns and modification on fire risk and structure damages by WUI fires in recent years. The automatic algorithms and workflow will be applied to other regions for larger scale applications.
We will be collaborating with data scientist and partnering with CalFire, Planet Labs, and a few counties with high WUI areas. The advanced deep learning-based data integration and object identification approaches, driven by multi-source satellite and social data, will refine the demographic and WUI characterization, providing critical geospatial data for land planning, exposure analysis, fire hazard risk assessment, and ultimately prioritization of fire planning. It will also expedite the labor-intensive post-disaster structure damage evaluation, identify the land use and urban development patterns that are less vulnerable for devastating fires, and evaluate the effectiveness of the fuel management within and nearby the WUI in reducing fire hazard.